Facebook AI Research Works On The Limitations Of Deep Learning Models
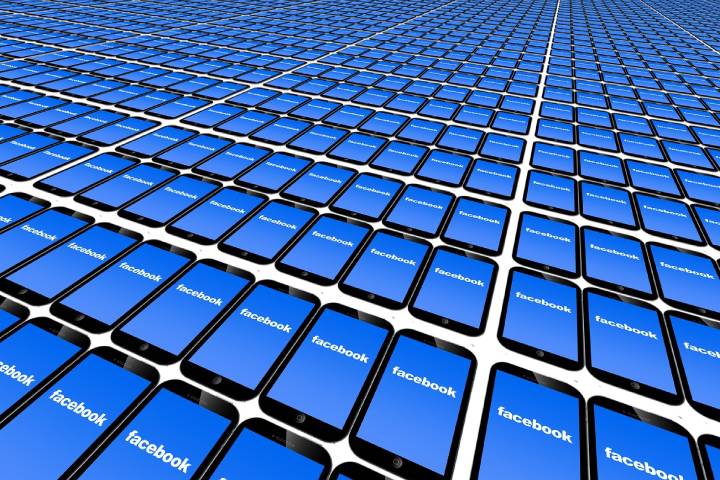
When we are before an image, human beings innately recognize each of the elements that make up the said image and its differentiating features based on the knowledge we have of the world around us. It is, therefore, that we can recognize when a cat or dog appears in an image, we realize it, as well as we can identify its breed, colour or that it has something that we have never encountered before, for example, a dog without a tail and lame.
Likewise, we can identify a Jack Russel, whether it appears in the profile image, from the front, upside down, jumping or even bathing on the beach. Thanks to Deep Learning models, Artificial Intelligence systems can interpret statistical patterns between pixels and tags, although they have some limitations to identify objects in their many natural variations correctly.
However, a human would know instantly for Artificial Intelligence models, factors such as colour, size, and perspective complicate a successful prediction. Likewise, Facebook AI has recently developed the idea of an “equivariant” exchange operator. This is a proof of concept for a workaround that could help deep learning models understand how an object can vary by mimicking the most common transformations.
The work Facebook AI is currently developing is mainly theoretical for the moment. However, it has broad potential for deep learning models, particularly in “computer vision”: the more interpretability and accuracy, the better the performance. Even when training on small data sets and more extraordinary ability to generalize.
The unravelling and its limitations in the current approach Unravelling is a solution to identify the natural variations that an object has. Its objective is to identify and distinguish between the factors of variation within the data. And that its inner workings are more understandable. Applying the ‘unravel’ in the example above to identify a dog in an image, a data set of dog images could be encoded into the pose, colour, and breed subspaces.
Discover factors of variation through “equivariant” operators However, what Facebook brings to this solution is that instead of restricting each transformation to one component of a representation, what if the changes could modify the entire presentation. Existing models are based on strict supervision, such as understanding the transformations of interest a priori and enforcing them in the model. The models can be trained to be equivalent to the changes of the subparts of an image, and, more importantly, the models could recombine the subparts when faced with unknown objects.
Image processing with AI model currently, a clear example of AI-based systems with deep learning models for image processing and object detection in images is our Visual Sensing system within the platform, based on intelligent content management. The Visual system Sensing can quickly detect both objects and people and make measurements based on images obtained by video cameras. In other words, the Visual Sensing system consists of using video cameras as sensors to address different application cases.
Allows you to do much more. It is a data platform that, under Artificial Intelligence models, has various functionalities for processing both images and videos, from searching for “what is said” in a video to finding and recognizing objects in pictures and generating tags. In addition to the search, functionality allows creating alerts on the things identified in the images and detecting similarity of photographs or otherwise, detecting duplications. Has dashboards with data visualizations for its analysis, measurement, and evaluation of the results quickly and straightforwardly.
Also Read: Machine Learning Is Coming As Close As Possible To Humans