Phase Change Memories for Recurrent Impulse Neural Networks
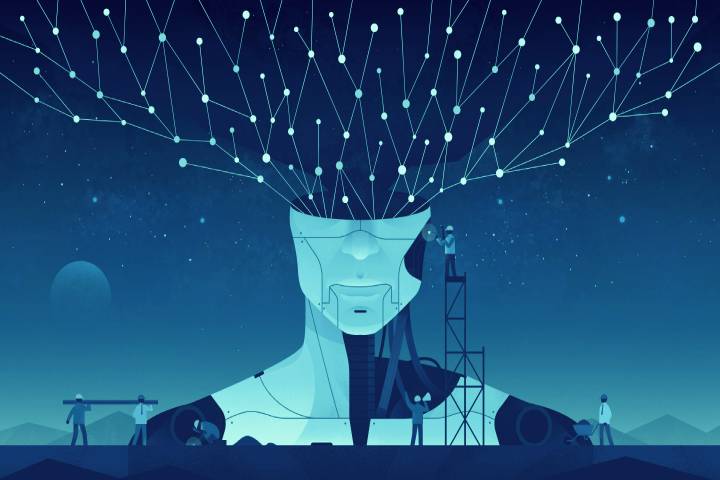
Swiss researchers have investigated the use of phase-shift memory technologies to improve in-memory computing used in recurrent impulse neural networks. According to their findings, the resistive SCM memory can be designed to train these types of networks more efficiently and online.
Impulse neural networks emulate the functioning of human neurons more realistically than classic artificial neural networks. This has led to the development of new impulse recurrent neural networks (RNN), whose nodes can work with larger data sets and relate to each other more dynamically and effectively. But this requires more efficient in-memory computing technologies built into each device that makes up an RNN.
According to experts, the greatest challenge in providing each node with its learning capabilities is the temporary allocation of credits and achieving more stable network dynamics, even when the weighting resolution of this allocation is limited. These are typical characteristics of RNNs, and it has been shown that these problems are accentuated by using memristive substrates for computation in local memory. Facing the Von-Newmann bottleneck in these architectures increases the variability in the calculation and the working memory of the recurrent impulse neural networks.
Conventional memristive, due to the substantial non-idealities of PCM. To solve this problem, a team of researchers at the University of Zurich has developed a differential architecture crossbar matrix simulation framework based on a more accurate and comprehensive phase change memory device (PCM) model. They have been able to train an impulse RNN by emulating the weights, using a recently proposed e-prop learning rule, which according to their appreciation, is close to the ideal conditions for synaptic updating, but which cannot be successfully implemented in memory.
In their simulations, they have compared the performance of their matrix with various widely adapted weight update schemes to resolve these device non-idealities. And they claim to have shown that it is possible to take advantage of the accumulation of gradients of phase change memory based on memristive substrates to achieve online training of recurrent impulse neural networks and significantly increase their efficiency.
Also Read: There Differences Between An ERP Cloud And An ERP SAAS