Why Machine Learning Projects Fail- 7 Reasons that can Take Your Efforts for a Ride?
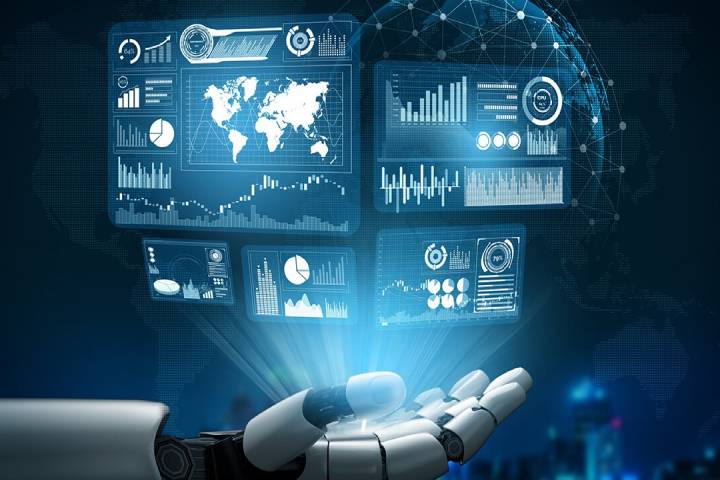
Your new Machine Learning project is about to fail. Yes, you read that right.
But don’t get to brooding. Not yet! Instead, it is more advisable to first understand the reasons why most other ML projects end up failing. Once you are aware of the possible and obvious pitfalls, you can simply go back to your project, eliminate them right away, and get your development back on track.
Also, as per VentureBeat, almost 87% of AI projects failed to make it through 2020, owing to a host of intrinsic factors. And as intelligent projects hinging on technologies like Computer Vision and Natural Language Processing cost a lot of money, failing isn’t always an option.
So before we talk about the ways to make your ML project a roaring success, let us delve right into the reasons that enhance fallibility:
Table of Contents
Lack of Expertise
Well, the first reason doesn’t need any validation. Machine learning projects require algorithms, data procurement, high-quality annotation, and other complex aspects taken good care of. Having an inexperienced team or data analytics person take care of these intricate ML concepts and developments is waiting for a mishap to happen. Final deployment, continuous monitoring, and successful predictive testing are far more critical and require the assistance of end-to-end service providers.
Subpar Data Volume and Quality
If you evaluate closely, the success of every ML project hinges on Data. Starting from data identification, aggregation, cleansing, augmentation to data labeling, the entire data-specific circle comprises 80% of the total time allocated to developing any machine learning model.
Image idea: Role of data and how 80% of the time is due to different data-specific tasks
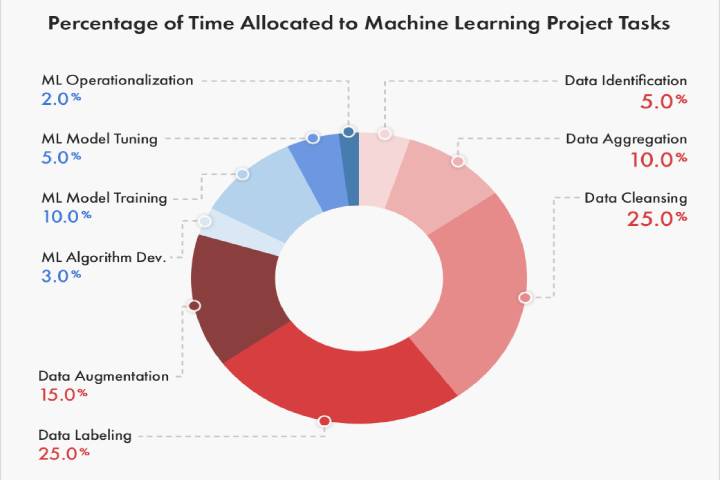
But then, most organizations, in an effort to launch the MVP at a lighting pace, end up compromising on data volume. The lack of diversity makes the models linear and far less intuitive than expected. Data quality also takes a hit if experienced AI training data collection services aren’t requested.
In the end, it is all about how well Data Science is implemented, and not many ML projects can get this part right.
Erroneous Labeling
Lack of properly annotated data can kill off even the most obvious projects. While labeled data can still be bad data at times, it actually covers the skill sets of your model provided the data collection vendors have done their job to perfection.
Did you know that almost 76% of global organizations end up annotating training data in-house, which stalls some of the more promising AI projects?
Organizations can scale beyond this issue either by outsourcing labeling to experienced service providers or by maintaining adequate training standards for the in-house data analysts, annotators, and scientists.
Lack of Proper Collaboration
Did you ever realize that AI and ML projects can even fail due to intrinsic factors? Surprised, right! Well, don’t be as every organization in charge of developing Machine Learning projects have BI specialists, Data Engineers, Data Scientists, DevOPs, and other professionals working in tandem. And finally, there is the core engineering team that takes the model to production.
However, if one segment fails to interact and collaborate with the other, data quality, volume, algorithms training data sets, testing sets, and other aspects can easily get compromised.
Dated Data Strategy
While a majority of concerns show up in between or at the fag end of the project conclusion, Data Strategy or the lack thereof is an issue that needs to be taken care of right at the onset. The factors included in a data strategy are often as follows:
- Total data requirement
- Can features be extracted from datasets
- What is the mode of data access?
- Does the procured data require cleaning, and if yes, to what end?
- Has the compliance been checked and verified?
- Pairing disparate datasets together for relevance and uniformity
Image idea: Volume of data preferred for organizations
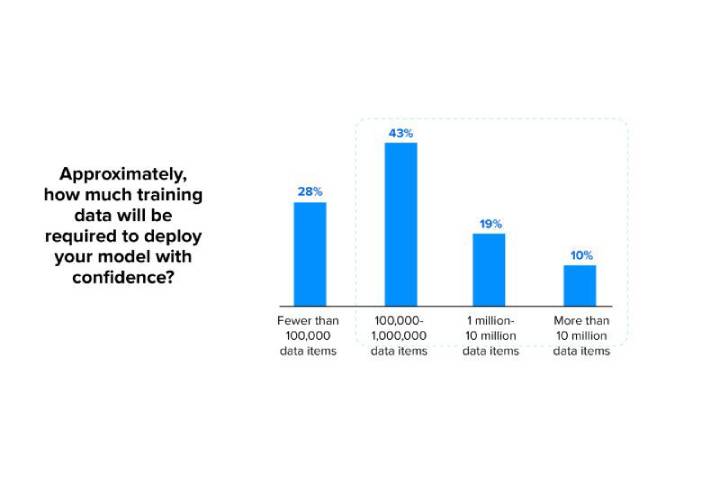
Most organizations planning to launch an ML project follow an obsolete data strategy that only talks about the sources and annotation techniques, which then results in project failure.
Absence of Efficient Leadership
It is important that the crux of the project is defined by an AI leader, who would even understand the practical implications of the product. Having a team of engineers, data scientists, and analysts handling the job is fine but if efficient leadership is missing, the project gets restricted to a mere confluence of technologies and datasets. In the end, it’s all about what difference the project makes and what problem it ends up solving, and these aspects can only be iterated by the business leader itself.
Unanticipated and Unpleasant Data Bias
To be honest, an intelligent ML model is all about automating tasks based on the data that has been fed to it. If the data collection, labeled and trained with is one-dimensional, chances of bias coming into the picture grow significantly. Unsure as to what data bias in AI and ML means? Well, here is an example.
Imagine there is a resume filtering product that picks the best candidate for the job by shortlisting 5 resumes out of 5000. However, the model ends up picking 5 male candidates when there were at least 35 highly qualified female candidates that should have been prioritized. On closer scrutiny, it is identified that the model and subsequent algorithms were fed with male-specific data sets, thereby leading to gender bias and instant elimination of female candidates.
Wrap-Up
While there can be many other reasons for an ML project to fail, these are 7 of the most common yet underrated reasons. And if you look closely, it all comes down to the procurement, usage, deployment, cleansing, annotation, implementation, and transformation of data. Also, as data forms the backbone of any machine learning campaign, it is advisable to onboard a credible end-to-end service provider to handle every aspect of the ML project with precision and accuracy.